Embracing AI in Healthcare: Transforming Patient Care Today
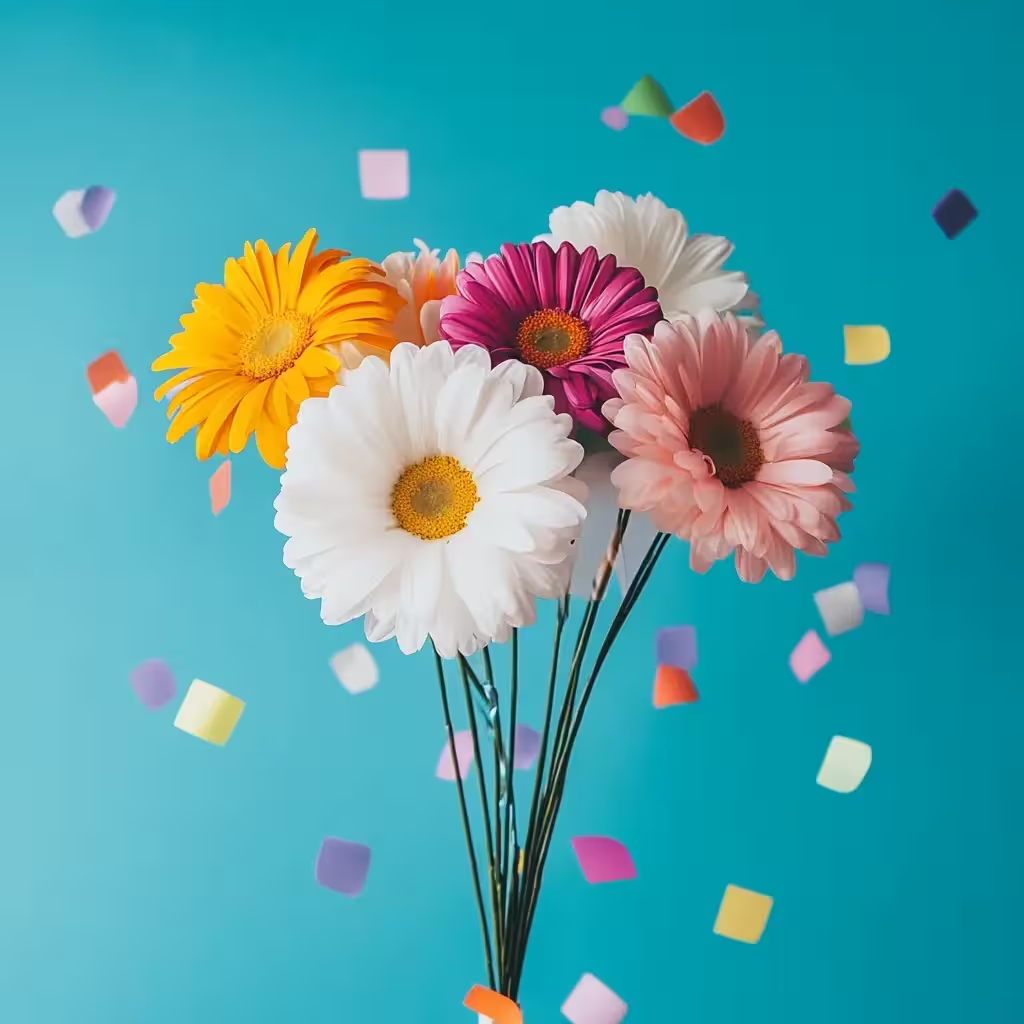
Embracing AI in Healthcare: Transforming Patient Care Today
Embracing AI: The Future of Healthcare Technology
Introduction
Artificial Intelligence (AI) is reshaping the landscape of healthcare, bringing in a new era of medical innovation and patient care. By using AI, healthcare professionals can provide more accurate, efficient, and personalized care to patients worldwide. The fast-paced growth of AI technologies—such as machine learning, deep learning, and natural language processing—is creating opportunities to improve diagnoses, treatment plans, and patient outcomes.
Adopting AI in healthcare technology is not just a choice but a necessity for tackling urgent issues like aging populations, rising costs, and the growing number of chronic diseases. Integrating AI solutions has the potential to enhance clinical decision-making, streamline operations, and ultimately lead to better health results.
In this article, we will explore:
- A comprehensive understanding of AI's role within the healthcare sector.
- Insights into how AI is transforming healthcare delivery.
- Key technologies that enable AI systems in medical settings.
- Steps for developing effective and reliable AI solutions tailored for healthcare.
- Ethical considerations and challenges in implementing AI in clinical practice.
- Future trends in AI-driven healthcare delivery.
- Success stories illustrating the impact of AI on patient care.
By delving into these topics, stakeholders can gain valuable knowledge on leveraging AI to drive meaningful change in healthcare.
Understanding Artificial Intelligence in Healthcare
Defining Artificial Intelligence and Its Relevance to Healthcare
Artificial Intelligence (AI) is the science and engineering of creating machines capable of performing tasks that typically require human intelligence. In the healthcare industry, AI's relevance is profound, offering the potential to revolutionize how medical services are delivered and improve patient outcomes. By processing vast amounts of data quickly and accurately, AI facilitates a more personalized approach to medicine, enhances diagnostic precision, and optimizes treatment plans.
Differentiating Machine Learning and Deep Learning
Two pivotal components of AI systems in healthcare are Machine Learning (ML) and Deep Learning (DL). Both play distinct yet interconnected roles:
- Machine Learning: This branch of AI involves algorithms that enable computers to learn from data without explicit programming. It encompasses various methodologies such as supervised learning, unsupervised learning, and reinforcement learning. ML applications in healthcare include predicting disease outbreaks, patient risk stratification, automating administrative tasks, and improving medical decision-making.
- Deep Learning: A subset of machine learning characterized by neural networks with many layers (deep neural networks). These networks excel in processing complex patterns in large datasets. In healthcare, DL is instrumental in image recognition tasks like radiology diagnostics where it identifies anomalies with high accuracy.
Overview of AI Systems Utilized in the Medical Field
The adoption of AI systems across the medical field is diverse and rapidly expanding:
- Diagnostic Imaging: AI-driven platforms analyze medical images with enhanced speed and accuracy. For instance, deep learning algorithms facilitate the automatic detection of conditions such as diabetic retinopathy from retinal scans.
- Virtual Health Assistants: These AI systems interact with patients through natural language processing to provide advice on symptoms or medication management, enhancing accessibility to healthcare information.
- Predictive Analytics: Leveraging machine learning algorithms, predictive analytics anticipate patient deterioration or readmission risks, allowing for preemptive clinical interventions.
- Robotic Processes: Robotic systems powered by AI assist surgeons during operations by providing real-time data analysis and precision control over surgical instruments.
The convergence of artificial intelligence with healthcare signifies a transformative era where AI not only augments human capabilities but fosters a new paradigm in patient care delivery. As we delve deeper into specific technologies enabling these advancements, understanding their integration into existing healthcare frameworks becomes crucial for maximizing their potential benefits.
Moreover, the successful implementation of these technologies often hinges on the organization's ability to adapt and evolve. This is where understanding the 14 key characteristics of high-performance organizations can be beneficial. By fostering a culture of excellence and driving sustained success, organizations can fully leverage the benefits offered by AI in healthcare.
The Role of AI in Transforming Healthcare Delivery
Healthcare delivery systems face numerous challenges, including rising costs, an aging population, and a growing burden of chronic diseases. These factors strain resources, affecting the quality and accessibility of care. Artificial Intelligence (AI) is poised to address these challenges by transforming healthcare delivery through digital health innovations and precision medicine.
Overcoming Current Challenges with AI
1. Resource Allocation
AI can optimize resource allocation by predicting patient admissions and discharges, allowing hospitals to manage staff and equipment more effectively. For instance, predictive analytics algorithms can forecast patient influx patterns, reducing wait times and improving operational efficiency.
2. Chronic Disease Management
Utilizing AI for continuous monitoring through wearable devices enables proactive management of chronic conditions like diabetes and heart disease. These insights allow healthcare providers to personalize treatment plans, thereby enhancing patient outcomes.
3. Data Integration
AI facilitates the integration of multi-modal data from electronic health records (EHRs), genomics, and imaging, offering a comprehensive view of a patient's health profile. This approach supports the development of personalized treatment strategies.
Enhancing Patient Care with AI Applications
AI's potential to improve patient care is evident in several successful applications across various medical fields:
1. Diagnostic Accuracy
Machine vision algorithms have shown remarkable success in diagnostic imaging platforms. Automated systems are now capable of identifying conditions such as diabetic retinopathy and certain cancers with precision that rivals human experts.
2. Virtual Health Assistants
These tools provide patients with 24/7 access to health information and support. For example, AI-powered chatbots like Babylon Health assist users in assessing symptoms and providing reliable medical advice, effectively acting as preliminary triage tools.
3. Surgical Precision
Robotic-assisted surgeries powered by AI enhance precision and reduce human error. Systems like the Da Vinci Surgical System integrate AI to guide surgeons during complex procedures, leading to better surgical outcomes and faster recovery times.
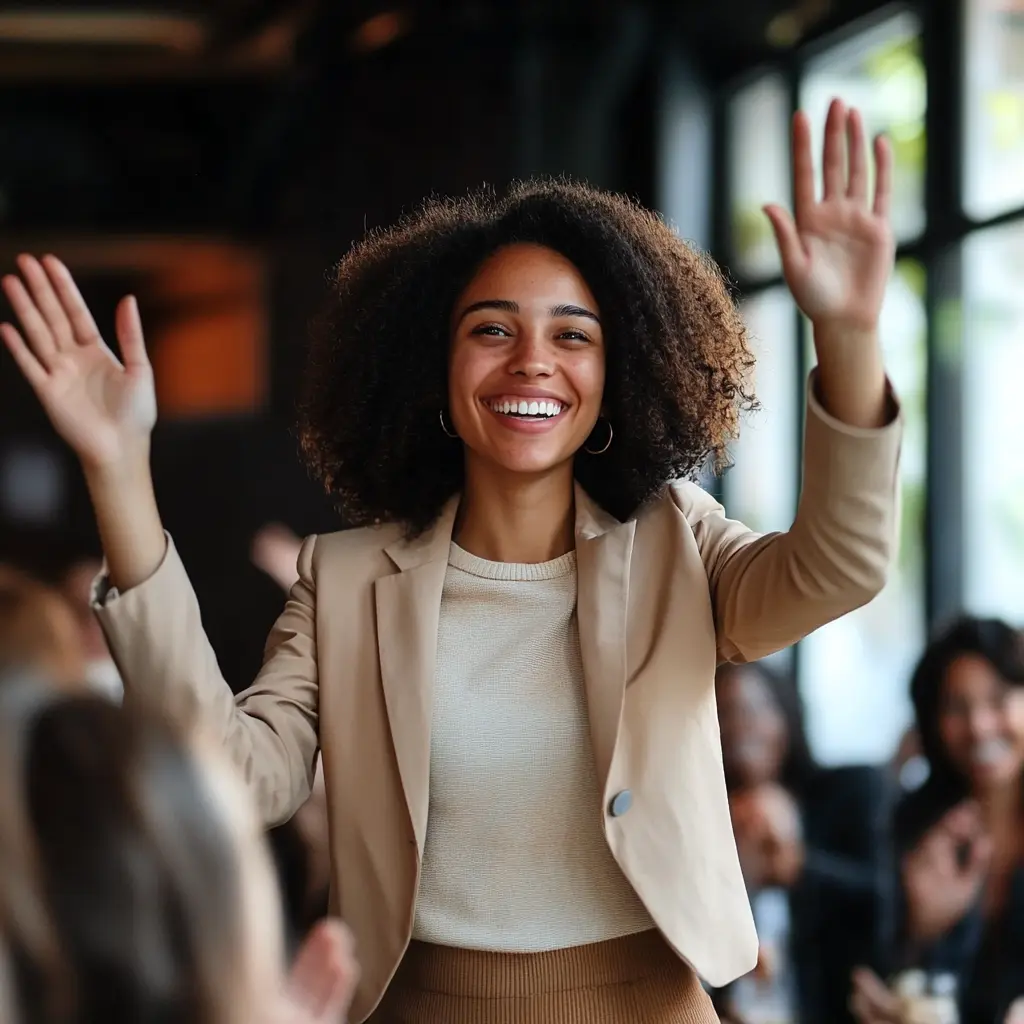
African American woman smiling in the workplace, expressing gratitude and positivity while embracing AI.
The Broader Implications of AI in Healthcare
AI's role extends beyond these examples as it continues to shape the future landscape of healthcare delivery. By integrating advanced technologies into clinical practice, healthcare systems are moving towards more efficient, precise, and accessible care solutions. This transformation promises not only to enhance patient experiences but also to redefine how healthcare is delivered globally.
Interestingly, the influence of AI is not restricted to the healthcare sector alone. It is also making significant strides in areas like HR, where its application is revolutionizing talent management processes such as hiring and performance management. As highlighted in a recent CPO PLAYBOOK podcast episode, bold leadership decisions are increasingly being informed by AI-driven data analysis, helping organizations navigate challenges like analysis paralysis.
Moreover, the concept of prompt engineering is gaining traction as businesses seek to empower their workforce with critical skills for creating effective AI prompts. This trend underscores the broader productivity potential that AI holds in the workplace, enabling employees to leverage technology for enhanced efficiency and professional growth.
In conclusion, whether it's in healthcare or HR
Key Technologies Enabling AI in Healthcare
Artificial intelligence (AI) is becoming a cornerstone of modern healthcare, driven by key technologies such as cloud computing and the Internet of Things (IoT). These technologies not only enhance the capabilities of AI systems but also ensure their safe and efficient integration into healthcare settings.
Cloud Computing: The Backbone of AI Systems
Cloud computing plays a crucial role in supporting AI systems by providing scalable resources and secure data storage. Healthcare organizations utilize cloud platforms to handle vast amounts of data generated daily—from patient records to diagnostic images—enabling complex AI algorithms to process this information efficiently.
Benefits of Cloud Computing in Healthcare AI
- Scalability and Flexibility: Cloud services offer elastic computing resources, allowing healthcare providers to scale their applications based on demand without significant capital investment in physical infrastructure.
- Data Security and Compliance: Leading cloud providers implement robust security measures and compliance frameworks (e.g., HIPAA in the US) that help protect sensitive health data while ensuring regulatory adherence.
- Cost Efficiency: By adopting cloud solutions, healthcare facilities can reduce IT maintenance costs, focusing resources on patient care rather than infrastructure management.
Innovations in Remote Monitoring through IoT
The synergy between IoT devices and AI is revolutionizing remote patient monitoring, offering new avenues for patient management and care delivery. IoT devices collect real-time health data, which AI analyzes to provide insights for timely interventions.
Impact of IoT on Patient Care
- Continuous Health Monitoring: Wearable devices like smartwatches track vital signs such as heart rate and activity levels. These IoT gadgets enable continuous monitoring outside traditional healthcare settings, providing valuable data for AI models to predict potential health issues.
- Enhanced Patient Management: IoT-enabled remote monitoring supports chronic disease management by facilitating early detection of anomalies. For instance, connected glucose monitors for diabetes patients allow for precise insulin dose adjustments based on real-time data analysis.
- Personalized Care Delivery: AI-driven insights from IoT device data enable tailored treatment plans. This personalization enhances patient outcomes by aligning medical interventions with individual health profiles.
Embracing AI for a Connected Future
Integrating cloud computing and IoT with AI fosters a more connected healthcare ecosystem. As these technologies evolve, they promise improved operational efficiencies, enhanced patient experiences, and better clinical outcomes. The convergence of these innovations marks a critical step toward a digitally enabled future where personalized medicine becomes the norm.
In addition to these advancements in healthcare, the principles of setting effective OKRs with AI tools like ChatGPT, as explored in our podcast episode with Enrique Rubio, are being increasingly adopted across various sectors including healthcare. This demonstrates the versatility of AI in not only enhancing patient care but also streamlining operational processes through strategic planning.
Moreover, the insights from our recent podcast episode about AI's role in innovating HR, highlight how similar technological advancements are reshaping human resource management within the healthcare sector.
Furthermore, understanding how businesses can harness AI technologies is essential for driving successful projects and shaping organizational transformation in this digital age. Lastly, exploring the potential of AI in workforce management, as discussed by Ali Nawab in our podcast episode, offers valuable insights into aligning talent strategies for financial success within the healthcare industry.
Developing Effective and Reliable AI Systems for Healthcare
Creating effective and reliable AI systems for healthcare involves a structured approach that addresses the specific needs of diverse healthcare settings. This process is not merely about technological innovation; it requires a comprehensive understanding of clinical environments, patient care protocols, and regulatory requirements. Here is a detailed exploration of the essential steps for developing trustworthy AI systems tailored to healthcare:
Steps to Building Trustworthy AI Systems
- Problem Definition
- Identify and articulate the specific healthcare challenges that the AI system will address. This involves understanding clinical workflows, patient care needs, and existing gaps in service delivery.
- Data Collection and Curation
- Gather high-quality, relevant data from multiple sources, ensuring it is representative of diverse patient populations. Data must be cleaned, anonymized, and formatted correctly to train machine learning models effectively.
- Algorithm Development
- Utilize state-of-the-art machine learning techniques to build models capable of recognizing patterns and making predictions based on the curated datasets. Emphasis should be placed on transparency and explainability of these algorithms to foster trust among healthcare providers.
- Validation and Testing
- Conduct rigorous validation through pilot studies to evaluate the statistical validity, clinical utility, and economic impact of the AI system. Continuous testing with real-world data helps refine model accuracy and reliability.
- Regulatory Compliance
- Ensure adherence to all relevant legal and ethical standards by engaging with regulatory bodies early in the development process. This includes compliance with HIPAA, GDPR, or other applicable regulations governing data privacy and security.
- Deployment Strategy
- Develop a deployment plan that considers integration into existing IT infrastructure within healthcare organizations. A phased rollout can help address initial technical challenges while facilitating user adaptation.
- Monitoring and Maintenance
- Implement continuous monitoring mechanisms to track system performance post-deployment. Regular updates and recalibrations are necessary to address emerging challenges and incorporate new medical knowledge or technologies.
Importance of Stakeholder Engagement
The development of AI systems for healthcare thrives on multidisciplinary collaboration:
- Clinical Stakeholders: Involve doctors, nurses, and allied health professionals who can provide insights into clinical needs and practical implementation challenges.
- Technical Experts: Collaborate with computer scientists and engineers specializing in AI technologies to ensure robust technical design.
- Operational Leadership: Engage healthcare administrators for strategic alignment with organizational goals.
- Patients & Advocacy Groups: Include voices from patients or their representatives to ensure solutions are patient-centered and equitable.
This collaborative approach not only enhances the relevance of AI solutions but also builds trust among end-users by demonstrating a commitment to addressing real-world healthcare issues comprehensively.
Ethical Considerations and Challenges in Implementing AI in Clinical Practice
The integration of Artificial Intelligence (AI) into clinical practice presents a myriad of challenges, particularly concerning data quality and access. The efficacy of AI systems hinges on robust, high-quality datasets.
Data Quality and Access Issues:
- Data Integrity: Ensuring the accuracy and consistency of data inputs is crucial. Incomplete or erroneous datasets can lead to flawed AI predictions, potentially compromising patient safety.
- Data Privacy: Protecting patient confidentiality is paramount. The use of sensitive health information necessitates stringent adherence to privacy regulations such as GDPR or HIPAA.
- Data Standardization: Variability in data formats across healthcare systems complicates AI implementation. Standardized data protocols are essential for seamless integration.
To address these data quality issues, leveraging insights from experts like Kyle Lagunas, who advocates for data quality and stakeholder alignment in performance management, could be beneficial.
Navigating technical infrastructure limitations also poses significant hurdles. Healthcare facilities often grapple with outdated IT systems that are ill-equipped to support advanced AI technologies.
- Infrastructure Upgrades: Updating legacy systems requires substantial investment and time, resources that may be scarce in underfunded healthcare settings.
- Interoperability: Ensuring that AI solutions can communicate effectively with existing medical software is critical for functionality and efficiency.
Regulatory Compliance remains a formidable challenge. The regulatory landscape for AI in healthcare is still evolving, making compliance a complex task.
- Ethical Standards: AI must be developed and deployed with ethical considerations at the forefront, ensuring fairness and avoiding biases that could exacerbate health disparities.
- Approval Processes: Navigating the approval processes for new AI applications involves understanding diverse regulatory requirements across different jurisdictions.
Ensuring ethical practices in AI deployment involves:
- Transparency: AI systems should offer explainable outcomes, allowing clinicians to understand and trust the decision-making process.
- Accountability: Clear guidelines must be established on who holds responsibility when AI-driven decisions impact patient care.
The successful implementation of AI in clinical practice demands a concerted effort to address these challenges. Stakeholders must prioritize ethical considerations alongside technological advancements to harness AI's full potential while maintaining patient trust and safety. Moreover, the incorporation of AI-Powered Job Skills into training programs could enhance workforce agility and readiness for this technological shift.

Doctors using advanced AI holographic displays for medical diagnostics in a futuristic high-tech healthcare setting.
Future Trends of AI in Healthcare Delivery
The future of AI in healthcare delivery is set to evolve significantly, changing how patients interact with medical professionals and receive care. The industry expects several transformative trends across short, medium, and long-term periods, with AI playing a crucial role.
Short-Term (0–5 Years): Augmented Telehealth
1. Augmented Telehealth Experiences
In the immediate future, telehealth will benefit from enhanced technologies such as virtual reality (VR) and natural language processing (NLP). VR can create immersive environments for patient consultations, enabling a more comprehensive understanding of conditions and treatments. NLP algorithms enhance communication by accurately interpreting and responding to patient inquiries, providing a more interactive and personalized experience.
2. Precision Imaging
AI's capabilities in image analysis will continue to improve diagnostic accuracy rapidly. Machine vision algorithms can identify subtle patterns in medical images that might be missed by human eyes, facilitating early detection and intervention.
Medium-Term (5–10 Years): Ambient Intelligence
1. Seamless Integration of Environments
The next phase involves ambient intelligence capabilities that integrate digital health interventions with physical environments. This technology allows healthcare systems to operate seamlessly within patients' lives, offering continuous monitoring and personalized feedback without disrupting daily activities. For instance, smart sensors embedded in homes could track vital signs or activity levels, alerting healthcare providers to any anomalies.
2. Enhanced Patient Engagement
Patients will experience increased autonomy over their health data and management through user-friendly platforms. These systems will provide real-time insights and recommendations based on individual health profiles, promoting proactive engagement in personal health management.
Long-Term (>10 Years): Autonomous Virtual Health Assistants
1. Revolutionizing Interactions
Looking further ahead, autonomous virtual health assistants are expected to play a crucial role in transforming patient-provider interactions. These AI-driven entities could manage routine check-ups, medication adherence, and even provide psychological support. By leveraging advanced machine learning models, these assistants would deliver tailored advice and interventions based on comprehensive patient data analytics.
2. Networked Healthcare Ecosystems
The vision includes a fully interconnected digital infrastructure where clinics, hospitals, caregivers, and patients communicate effortlessly via AI-powered platforms. This model supports holistic care delivery by ensuring information continuity across different care settings.
Embracing AI along this evolutionary path promises not only to enhance efficiency but also to elevate the quality of care provided globally. Each phase presents unique opportunities for innovation while addressing existing challenges within the healthcare landscape.
Case Studies Highlighting Successful Implementation of AI Solutions in Healthcare
DeepMind's AlphaFold: A Game-Changer in Protein Structure Prediction
DeepMind's AlphaFold project is a groundbreaking achievement in artificial intelligence, especially in the field of biotechnology. It uses advanced deep learning techniques to predict protein structures with unmatched accuracy. This breakthrough solves a major problem in biology — understanding the 3D structure of proteins, which is essential for understanding their function and role in diseases.
Impact
The ability to predict protein structures so accurately speeds up drug discovery processes and helps develop new treatments. Researchers can now find potential drug targets more efficiently, saving time and money compared to traditional experimental methods.
Application
In practical terms, researchers around the world are already using AlphaFold’s models to gain new insights into complex biological processes, leading to innovations in personalized medicine.
Automated Diagnostic Imaging Platforms: Transforming Disease Detection
Automated diagnostic imaging platforms are revolutionizing medical diagnostics through the use of machine vision algorithms. These platforms leverage AI-powered technologies to improve image analysis capabilities across various medical specialties such as radiology, dermatology, and pathology.
Use Cases
- Diabetic Retinopathy Screening: Countries like the USA, Singapore, Thailand, and India have implemented automated AI algorithms for diabetic retinopathy screening. These algorithms significantly enhance early detection rates, preventing severe complications through timely intervention.
- Radiotherapy Planning: Microsoft's InnerEye technology exemplifies how AI can optimize radiotherapy planning. By employing machine learning models, InnerEye reduces preparation time by up to 90%, enhancing both efficiency and accuracy in treatment delivery.
Precision Therapeutics: Advancing Targeted Treatment Strategies
The integration of AI into therapeutic development processes has led to significant advances in precision therapeutics. Technologies such as immunomics and synthetic biology are utilizing AI to create targeted preventive strategies and improve clinical trial designs.
AI-Driven Drug Discovery
By analyzing large datasets consisting of genomic, proteomic, and metabolomic information, AI enables rapid identification of promising drug candidates. This approach not only speeds up the drug discovery process but also enhances the precision of treatment plans tailored to individual patient profiles.
These case studies highlight the transformative potential of current applications of AI in healthcare. From speeding up scientific research to optimizing clinical practices, these examples demonstrate how AI is reshaping boundaries within healthcare systems. The ongoing evolution holds the promise of continuous advancements that will further integrate AI solutions into everyday medical practices.
Conclusion
The integration of AI into healthcare systems marks a significant turning point, with the potential to reshape patient care and improve operational efficiency. Using technology for better healthcare outcomes is not just an option but a requirement in light of growing global health issues.
Key players such as clinicians, technologists, and policymakers have the power to fully realize AI's potential. By working together, these diverse groups can create groundbreaking solutions that improve patient care experiences. Collaborative efforts will lead to the creation of systems where human expertise is enhanced by AI abilities, resulting in more precise diagnoses, tailored treatments, and streamlined healthcare delivery.
A vision of a digitally enabled future is within reach—a future where all health services are interconnected through advanced technological solutions. This interconnectedness promises a seamless experience for patients and providers alike, breaking down barriers and promoting ongoing improvement.
The path towards adopting AI in healthcare requires dedication from all parties involved. By utilizing state-of-the-art technologies, we can make healthcare delivery transformation achievable, setting new benchmarks for what's possible in medical innovation and patient care. This change is particularly important in the Life Sciences Industry, where organizations are increasingly using AI to enhance human capital strategies and achieve sustainable growth.